Python异步: 从入门到入___
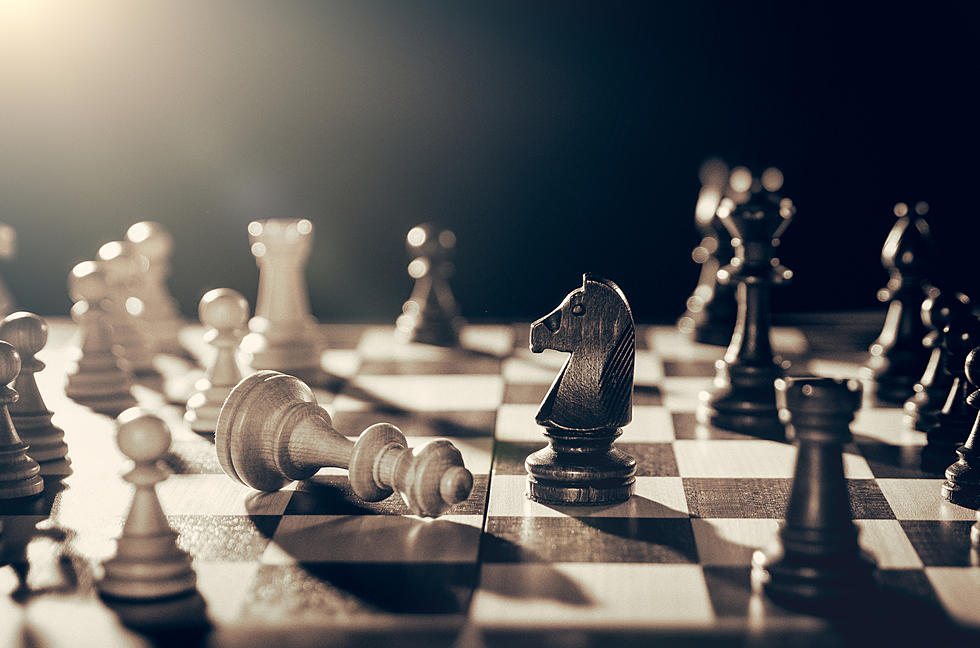
异步真的很快,至于入什么也不是我说了算,看您是哪种 * 操作了。
🍺本篇文章大约需要4分钟来阅读,届时你或许将学到:
- 异步原理简要
asyncio
的基础用法 (3.7+特性)Coroutines
、Task
、Future
- 编写你的第一段异步请求
0x01 生活中的异步
就好比人活着就已经是种异步行为了,虽然我们是单线程的,但你可以同时做多件事,对吧?
例如现在有三件事需要你完成,分别为:
- 洗衣服 30m
- 看剧 20m
- 烧水 2m
你应该不会需要,52分钟吧?或许有人会问 多人运动
算不算异步,抱歉啊那是 并行
0x02 为何用异步
通过 0x01 我们不难知道,将事件集合一个接一个得进行会导致耗时过长
而异步有着良好的时间管理,并通过 暂停A去做B 并来回切换 的方式,将节省大量时间。
0x03 Coroutines
接下来我们看一组异步代码运行0x01
的三个任务,并通过 livepython
来监测它的工作方式
按照同步的方式,他们将依次执行。而转换成异步后,可以明显看出执行步骤将自动切换
生成器与之较为相似,但非本章重点,故略过
然后我们参照该动画讲解异步,A、B、C
方法均为 coroutines
即协程,与普通定义的方法很像,不一样的是它们
-
可将方法暂停
-
需通过
async def
前缀来定义 -
需运行在事件循环
loop
中
0x04 Async & Await
这俩关键字为Python3.5新特性,使得异步写法愈发简洁,相当于将原先的语法进行了替换
-
@asyncio.coroutine
变为async
-
yield from
变为await
await 的作用为暂停方法,并交出控制权限,直到后续表达式执行完毕才继续进行
旧版写法
import asyncio
@asyncio.coroutine
def A():
print('A start')
yield from asyncio.sleep(10)
print('A end')
不过如上写法已经不太推荐,故在python3.8
中出现会有DeprecationWarning
的警告
0x04 Task & Future
Task
是通过打包协程所生成的,而它的存在意义就是用于任务并发。
打包的方法也非常简单,即如下代码中的asyncio.create_task
过程
import asyncio
async def func(i):
...
task = asyncio.create_task(func(10))
Future
简单的理解就是未完成的任务集合,它的意义在于方便我们对任务集的管控。我们可以从中看到任务集状态,及结果
是时候讲讲并发了,也就是将多个任务交由 asyncio.gather
方法处理
async def main():
return await asyncio.gather(
func(10),
func(13),
...
)
asyncio.run(main())
0x05 Awaitable
我们来梳理一下他们之间的关系,并画了张图
-
普通方法通过
async
关键字可以变成协程(coroutine)
-
coroutine
可通过asyncio.create_task
将其转为任务(task)
-
task
继承自(future)
-
coroutine
、task
和future
对象皆为 可等待对象(awaitable)
-
awaitable
对象都只能在coroutine
中进行await
操作 -
awaitable
对象通过asyncio.gather
将获得一个future
继承
0x06 并发请求
如果不实践一下的话,可能上面的内容就当小说看了。于是我们设计了如下入门demo来并发请求,这里我们就需要借助大名鼎鼎的异步请求库 aiohttp
import asyncio
import aiohttp
我们预设一个方法用于输入事件时间
from datetime import datetime
current = lambda: datetime.now()
并且准备好需要访问的站点
urls = [
'https://hulu.com',
'https://google.com',
'https://netflix.com',
'https://youtube.com'
]
然后我们编写单任务请求方法及多任务并发方法
async def fetch(url):
print(f"[{current()}]start {url}")
async with aiohttp.ClientSession() as session:
resp = await session.get(url)
print(f"[{current()}]end {url}")
return resp
async def run_all():
return await asyncio.gather(
*list(map(fetch,urls))
)
results = asyncio.run(run_all())
然后运行整个代码,这里需借助 timeit
来统计任务用时
timeit python3 test_asyncio.py
最后我们看到日志缓缓输出,这里就不缓缓了。
[2020-05-06 22:31:47.055686]start https://hulu.com
[2020-05-06 22:31:47.077206]start https://google.com
[2020-05-06 22:31:47.078004]start https://netflix.com
[2020-05-06 22:31:47.078528]start https://youtube.com
[2020-05-06 22:31:47.467144]end https://youtube.com
[2020-05-06 22:31:47.495745]end https://google.com
[2020-05-06 22:31:49.468241]end https://netflix.com
[2020-05-06 22:31:49.622931]end https://hulu.com
python3 test_asyncio.py 0.64s user 0.14s system 23% cpu 3.254 total
所有任务几乎被同时启动并处理,符合我们的预期效果